What does MLLS mean in EDUCATIONAL
MLLS is a specialized field that combines machine learning techniques with biological, medical, and healthcare data to improve healthcare outcomes. It enables researchers and healthcare professionals to analyze complex data, identify patterns, and make predictions to enhance disease diagnosis, treatment planning, and drug discovery.
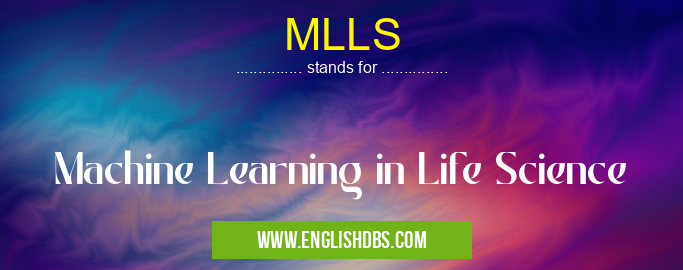
MLLS meaning in Educational in Community
MLLS mostly used in an acronym Educational in Category Community that means Machine Learning in Life Science
Shorthand: MLLS,
Full Form: Machine Learning in Life Science
For more information of "Machine Learning in Life Science", see the section below.
» Community » Educational
Key Aspects of MLLS
- Data-intensive: MLLS heavily relies on massive data sets generated from various sources such as high-throughput genomics, proteomics, and imaging.
- Algorithm development: MLLS involves developing and applying machine learning algorithms to analyze these large-scale data sets and extract meaningful patterns.
- Interdisciplinary: MLLS is a highly interdisciplinary field that combines expertise from computer science, statistics, and life sciences.
Applications of MLLS
- Drug discovery: Identifying potential drug targets, optimizing drug design, and predicting drug response.
- Disease diagnosis: Developing diagnostic tools for early detection and personalized medicine.
- Biomarker discovery: Identifying biomarkers for disease diagnosis, prognosis, and treatment monitoring.
- Genome analysis: Understanding gene regulation, genetic variation, and disease susceptibility.
- Protein structure prediction: Predicting the 3D structure of proteins from their amino acid sequence.
Benefits of MLLS
- Improved accuracy and efficiency: MLLS algorithms can analyze vast data sets and identify patterns that are difficult or impossible to detect manually.
- Precision medicine: MLLS enables personalized treatment plans based on individual patient profiles.
- Accelerated discovery: MLLS can significantly speed up the process of scientific discovery by automating data analysis and hypothesis testing.
Essential Questions and Answers on Machine Learning in Life Science in "COMMUNITY»EDUCATIONAL"
What is Machine Learning in Life Science (MLLS)?
What are the key applications of MLLS?
MLLS has numerous applications, including:
- Disease diagnosis: Identifying patterns in patient data to diagnose diseases more accurately and in early stages.
- Treatment personalization: Tailoring treatments to individual patients based on their unique genetic, medical, and environmental factors.
- Drug discovery: Accelerating the identification and development of new drugs by analyzing vast amounts of biological and clinical data.
- Healthcare system optimization: Improving operational efficiency, resource allocation, and the delivery of healthcare services through data-driven insights.
What types of data are used in MLLS? A: MLLS utilizes various types of data, including: - Electronic health records (EHRs): Patient medical history, demographics, diagnoses, medications, and outcomes. - Genomic data: DNA and RNA sequencing information, providing insights into genetic predispositions and disease susceptibility. - Medical imaging data: X-rays, MRI scans, and CT scans, facilitating disease detection and monitoring. - Environmental dat
MLLS utilizes various types of data, including:
- Electronic health records (EHRs): Patient medical history, demographics, diagnoses, medications, and outcomes.
- Genomic data: DNA and RNA sequencing information, providing insights into genetic predispositions and disease susceptibility.
- Medical imaging data: X-rays, MRI scans, and CT scans, facilitating disease detection and monitoring.
- Environmental data: Exposure to pollutants, dietary habits, and lifestyle factors, influencing health outcomes.
What challenges are associated with MLLS?
MLLS faces some challenges, such as:
- Data diversity and complexity: Managing and analyzing large, heterogeneous datasets from multiple sources requires advanced computational techniques.
- Ethical considerations: Ensuring data privacy, protecting patient confidentiality, and addressing bias in algorithms is crucial.
- Regulatory compliance: Adhering to stringent regulatory guidelines to ensure the validity and trustworthiness of MLLS models.
- Lack of skilled workforce: Training and retaining professionals with expertise in both machine learning and life sciences is essential.
What are the future trends in MLLS?
MLLS is rapidly evolving, with emerging trends including:
- Deep learning and artificial intelligence (AI): Leveraging advanced algorithms for more accurate predictions and insights.
- Integration with wearable devices and sensors: Collecting real-time patient data for personalized monitoring and interventions.
- Precision and personalized medicine: Utilizing MLLS to tailor treatments to individual patient needs and improve healthcare outcomes.
- Translational research: Bridging the gap between MLLS research and clinical practice for real-world applications.
Final Words: MLLS is a transformative field that is revolutionizing the life sciences. By harnessing the power of machine learning, researchers and clinicians can gain unprecedented insights into biological systems, leading to improved diagnostics, treatments, and personalized medicine.