What does FBPN mean in UNCLASSIFIED
FBPN stands for Feature Balanced Pyramid Network, a deep learning technique used to improve the accuracy and robustness of computer vision systems. FBPN is a novel approach that combines the benefits of feature extraction from deep networks with the increased performance achievable from data augmentation and transfer learning approaches. By combining these methods, FBPN can detect and classify objects with greater accuracy than traditional convolutional neural network (CNN) methods.
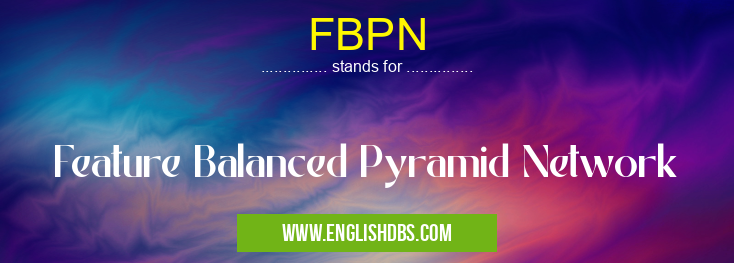
FBPN meaning in Unclassified in Miscellaneous
FBPN mostly used in an acronym Unclassified in Category Miscellaneous that means Feature Balanced Pyramid Network
Shorthand: FBPN,
Full Form: Feature Balanced Pyramid Network
For more information of "Feature Balanced Pyramid Network", see the section below.
Overview
FBPN uses a hierarchical architecture, consisting of four stages that process images from raw input to final classification. An image is fed into the first stage, where features are extracted using CNNs. The extracted features are then passed on to subsequent stages in the hierarchy, where they are processed further before finally being classified into one of several categories or classes by the last stage in the pipeline. Through its combination of feature extraction and data augmentation techniques, FBPN is able to build strong models that can accurately identify objects with high confidence levels even under difficult conditions such as varying lighting, camera angles or occlusions.
Benefits
The primary benefit of using FBPN compared to conventional CNN approaches is its ability to leverage new data sources with existing knowledge while maintaining high performance levels across all categories of an object recognition task. FBPN is also beneficial because it reduces training time by sharing weights across multiple layers in its architectures while still performing image processing tasks efficiently. Additionally, by utilizing transfer learning techniques such as pre-trained models and auxiliary networks, FBPN can quickly learn from existing datasets without needing to retrain each time new datasets become available.
Essential Questions and Answers on Feature Balanced Pyramid Network in "MISCELLANEOUS»UNFILED"
What is a Feature Balanced Pyramid Network?
A Feature Balanced Pyramid Network (FBPN) is a type of convolutional neural network architecture designed for object detection and segmentation tasks. It uses a pyramidal set of layered features to combine the low-level qualities of images such as edges and textures with higher-level, contextual information. The goal of FBPN is to create an output which accurately represents the objects in an image.
How does Feature Balanced Pyramid Network work?
Feature Balanced Pyramid Networks use the process of feature pyramid extraction to construct several layers of features from an input image. This involves extracting small patches from both lower and higher resolution versions of the image, so that there is a blend between local details and global context from various scales. Each layer of feature extractions then gets combined into a single representation, allowing objects in an image to be accurately identified by the CNN.
What advantages does FBPN provide?
FBPNs offer several advantages over traditional CNN-based object detection architectures. First, it has better robustness to scale variance, meaning it can maintain high accuracy even when the size of objects in an image changes dramatically. Additionally, FBPNs are faster than most other algorithms because they need to run fewer operations on fewer layers. Finally, since they merge both local and global information about an image together, FBPNs have been shown to produce more accurate results compared to other models at detecting multiple objects simultaneously.
Are there any limitations of using an FBPN?
Some drawbacks associated with using an FBPN include cost effectiveness and memory footprint. As each layer requires separate feature extraction operations, they can be significantly more expensive than other CNN architectures which perform feature extraction on a single layer only. Additionally, due to their complex structure, many FBPN networks can require relatively large amounts of memory allocation at runtime which can limit their usage depending on hardware capacity restrictions.
What types of applications can benefit from using Feature Balanced Pyramid Networks?
FBPNs are useful for any applications that require object detection or segmentation as part of their workflow such as autonomous vehicle navigation systems or computer vision tasks related to medical imaging analysis. In addition, they excel at multi-object tracking tasks like people counting or video surveillance where robust accuracy is often necessary under conditions with varying levels of scale variance or clutter in the scene being observed by the model.
Is it advisable to use Feature Balanced Pyramid Networks for classification tasks?
While FBPNS are powerful for detecting multiple objects within images or videos, they are not typically used for general classification tasks such as recognizing handwritten digits or labeling images based on their contents (i.e., categorization). For these types tasks simpler single layer CNN architectures are usually preferred due their low cost and efficiency.
Final Words:
In summary, Feature Balanced Pyramid Networks (FBPN) has emerged as an effective approach for improving object recognition systems through feature extraction and data augmentation when compared to conventional CNN approaches. By leveraging transfer learning techniques such as pre-trained models or auxiliary networks alongside their own hierarchal architectures, FBPN can quickly create powerful models that can accurately recognize objects even under challenging conditions like varying lighting or camera angles. With its combination of efficient training times and strong performance across all types of image recognition tasks, FBPN promises to be a powerful tool for use in future computer vision systems.