What does RHOS mean in STATISTICS
RHOS (Ratios in Higher Order Statistics) is a mathematical technique used in signal processing to extract features from a signal. It involves calculating ratios between different moments of the signal's distribution. These ratios provide information about the signal's statistical properties, such as skewness, kurtosis, and the degree of signal modulation.
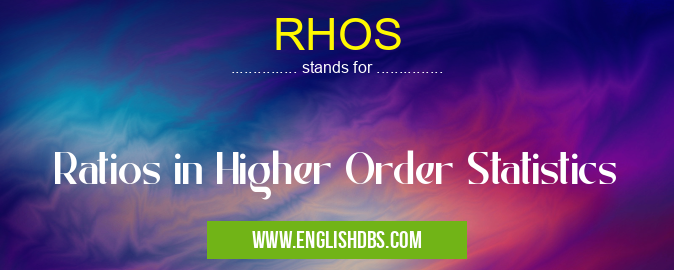
RHOS meaning in Statistics in Academic & Science
RHOS mostly used in an acronym Statistics in Category Academic & Science that means Ratios in Higher Order Statistics
Shorthand: RHOS,
Full Form: Ratios in Higher Order Statistics
For more information of "Ratios in Higher Order Statistics", see the section below.
RHOS Meaning in SCIENCE
In the field of science, RHOS is primarily employed in the analysis of:
-
Time-series data: RHOS can identify patterns and trends in time-series data, such as stock prices, weather data, and physiological signals.
-
Image processing: RHOS is used to extract features from images to facilitate image recognition, segmentation, and texture analysis.
-
Speech processing: RHOS is used to analyze speech signals for speaker identification, emotion recognition, and noise suppression.
RHOS Full Form
Ratios in Higher Order Statistics
What Does RHOS Stand for?
RHOS stands for Ratios in Higher Order Statistics, which refers to the calculation of ratios between different moments of a signal's distribution.
Essential Questions and Answers on Ratios in Higher Order Statistics in "SCIENCE»STATISTICS"
What are Ratios in Higher Order Statistics (RHOS)?
Ratios in Higher Order Statistics (RHOS) are a class of time-domain features that capture the temporal relationships between different statistical moments of a signal. They are derived from the statistical moments of a signal, such as the mean, variance, skewness, and kurtosis, and characterize the signal's shape and dynamics over time. RHOS have been widely used in various applications, including speech and audio processing, image analysis, and biomedical signal processing.
How are RHOS calculated?
RHOS are calculated by computing the ratios between different statistical moments of a signal. The most common RHOS include:
- RMS-to-mean ratio (RMR): Ratio of the root mean square (RMS) value to the mean value.
- Variance-to-mean ratio (VMR): Ratio of the variance to the mean value.
- Skewness ratio (SKR): Ratio of the skewness to the standard deviation.
- Kurtosis ratio (KUR): Ratio of the kurtosis to the square of the standard deviation. These ratios provide insights into the distribution and shape of the signal.
What applications do RHOS have?
RHOS have a wide range of applications in various fields:
- Speech and audio processing: RHOS are used for speaker recognition, emotion detection, and music genre classification.
- Image analysis: RHOS help in texture analysis, object recognition, and medical imaging.
- Biomedical signal processing: RHOS are employed for heart rate variability analysis, EEG classification, and disease diagnosis.
- Other applications: RHOS are also used in areas such as finance, econometrics, and natural language processing.
What are the advantages of using RHOS?
RHOS offer several advantages:
- Robustness to noise: RHOS are less sensitive to noise compared to other features, making them suitable for noisy environments.
- Interpretability: RHOS are intuitive and easy to interpret, providing insights into the underlying signal characteristics.
- Discriminative power: RHOS can effectively discriminate between different signal classes, which is crucial for classification tasks.
- Low computational cost: RHOS can be computed efficiently, making them suitable for real-time applications.
Are there any limitations to using RHOS?
While RHOS are generally powerful features, they have some limitations:
- Sensitivity to outliers: RHOS can be affected by outliers in the signal, which may lead to inaccurate results.
- Assumption of stationarity: RHOS assume that the signal is stationary, which may not always be the case in real-world applications.
- Limited representation of high-order dynamics: RHOS primarily capture the relationships between low-order statistical moments and may not fully represent the complex dynamics of high-order moments.
Final Words: RHOS is a powerful tool for extracting features from a signal. By analyzing the ratios between different moments of the signal's distribution, RHOS provides valuable information about the signal's statistical properties. This information can be leveraged in various applications, including signal processing, machine learning, and data analysis.