What does NNV mean in UNCLASSIFIED
NNV (Neural Network Verification) is a crucial aspect of artificial intelligence (AI) that involves assessing the reliability and accuracy of neural networks. These networks are complex algorithms used in a wide range of applications, including image recognition, natural language processing, and machine learning. Verifying the correctness and safety of neural networks is essential to ensure their trustworthiness and prevent potential risks.
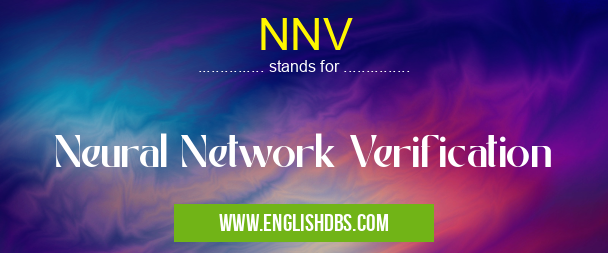
NNV meaning in Unclassified in Miscellaneous
NNV mostly used in an acronym Unclassified in Category Miscellaneous that means Neural Network Verification
Shorthand: NNV,
Full Form: Neural Network Verification
For more information of "Neural Network Verification", see the section below.
Methods of NNV
Various techniques are used for NNV, including:
- Formal Verification: This involves using mathematical methods to prove that a neural network meets certain specifications.
- Testing: By providing a diverse set of inputs to the network and evaluating the outputs, its behavior can be analyzed.
- Symbolic Execution: This method involves exploring the different paths a neural network can take during execution, identifying potential errors.
Importance of NNV
NNV is crucial for several reasons:
- Safety: Verifying neural networks is critical in safety-critical applications, such as autonomous vehicles or medical diagnosis, where incorrect outputs could have catastrophic consequences.
- Trustworthiness: Establishing the reliability and accuracy of neural networks increases user confidence in AI systems.
- Debugging: NNV helps identify and address errors in neural network models, improving their performance and resilience.
Essential Questions and Answers on Neural Network Verification in "MISCELLANEOUS»UNFILED"
What is Neural Network Verification (NNV)?
NNV is the process of formally proving that a neural network (NN) meets certain specifications or properties. It aims to ensure that NNs behave as intended and produce reliable results, particularly in safety-critical applications.
Why is NNV important?
NNV is crucial for increasing trust in NNs, especially when they are used in areas where incorrect predictions can have severe consequences. It helps establish confidence that NNs operate within defined boundaries and make decisions based on sound principles.
What are the challenges in NNV?
NNV poses several challenges due to the complexity and black-box nature of NNs. These include handling non-linearity, ensuring robustness to adversarial examples, and verifying NNs trained on large datasets.
What techniques are used for NNV?
Various techniques are employed in NNV, including:
- Formal methods: Using mathematical techniques to prove properties about NNs.
- Symbolic execution: Analyzing NNs by simulating their execution and exploring different paths.
- Bounded verification: Establishing upper and lower bounds on NN outputs to ensure compliance with specifications.
- Adversarial testing: Generating inputs that challenge NNs and assess their robustness.
What are the limitations of NNV?
While NNV provides valuable insights, it has limitations:
- Scalability: Verification techniques may not be applicable to large-scale NNs.
- Coverage: Verifying only a subset of behaviors may not guarantee the overall correctness of NNs.
- False positives: NNV methods may sometimes report errors in NNs that are not actual issues.
Final Words: Neural Network Verification is a fundamental aspect of AI that ensures the reliability and safety of neural networks. By employing various techniques, NNV provides a rigorous approach to assess the correctness and trustworthiness of these complex algorithms. As AI continues to advance, NNV will play an increasingly vital role in ensuring the responsible development and deployment of AI systems.