What does DSNN mean in UNCLASSIFIED
Dynamic Synapse Neural Network (DSNN), a groundbreaking concept in the realm of artificial intelligence (AI), draws inspiration from the intricate workings of the human brain. It introduces a novel approach to neural network architecture, mirroring the dynamic adaptability and learning capabilities exhibited by biological neural networks.
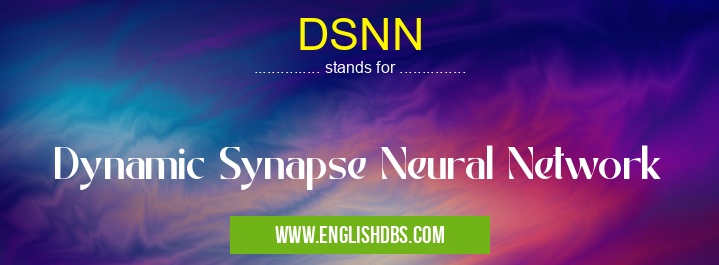
DSNN meaning in Unclassified in Miscellaneous
DSNN mostly used in an acronym Unclassified in Category Miscellaneous that means Dynamic Synapse Neural Network
Shorthand: DSNN,
Full Form: Dynamic Synapse Neural Network
For more information of "Dynamic Synapse Neural Network", see the section below.
Meaning of DSNN
DSNN stands for Dynamic Synapse Neural Network, emphasizing the fundamental characteristic that sets it apart: the dynamic nature of its synaptic connections. These connections, akin to the synapses in the human brain, possess the remarkable ability to strengthen or weaken over time, facilitating the acquisition of knowledge and the adaptation to changing environments.
Key Features of DSNN
-
Synaptic Plasticity: Unlike traditional neural networks, DSNNs feature dynamic synaptic connections that can adjust their strength based on the input data and learning experiences. This plasticity enables the network to continuously refine its knowledge and adapt to novel situations.
-
Online Learning: DSNNs excel in online learning scenarios, where data is presented sequentially and the network must adapt incrementally. They can effectively learn from streaming data, making them ideal for real-time applications such as natural language processing and autonomous systems.
-
Robustness: DSNNs exhibit enhanced robustness compared to static neural networks. The dynamic nature of their synapses allows them to tolerate noisy or corrupted data, ensuring reliable performance even in challenging environments.
Applications of DSNN
The versatility of DSNNs has led to their widespread adoption in various applications, including:
- Image Recognition
- Natural Language Processing
- Autonomous Driving
- Machine Translation
- Financial Forecasting
Essential Questions and Answers on Dynamic Synapse Neural Network in "MISCELLANEOUS»UNFILED"
What is a Dynamic Synapse Neural Network (DSNN)?
A DSNN is a type of artificial neural network that mimics the brain's ability to change and adapt its connections over time. It uses a dynamic learning algorithm that allows it to modify its synaptic weights based on the input data, making it suitable for tasks that require continuous learning and adaptation.
What are the key features of a DSNN?
Key features include:
- Dynamic Synaptic Weights: Synaptic weights can change over time based on input data, enabling the network to adapt and learn.
- Learning Algorithm: Uses a learning algorithm that allows for continuous learning and adaptation to changing inputs.
- Plasticity: Can modify its connections and structure in response to new information, similar to the brain's neuroplasticity.
What are the benefits of using a DSNN?
Benefits include:
- Adaptive Learning: Can automatically adjust its parameters based on input data, making it suitable for environments with changing or uncertain inputs.
- Continuous Learning: Can learn and adapt over time without the need for explicit retraining, enabling it to handle evolving data patterns.
- Flexibility: Can be customized to specific tasks by adjusting the learning algorithm and network architecture.
What are the potential applications of DSNNs?
Potential applications include:
- Real-time Prediction: Predicting outcomes based on continuously changing data, such as in financial markets or weather forecasting.
- Adaptive Control: Controlling systems that require constant adjustments based on changing inputs, for example, in robotics or automated vehicles.
- Time-Series Analysis: Modeling and forecasting time-varying data, such as in demand forecasting or anomaly detection.
How does a DSNN differ from a traditional neural network?
Traditional neural networks typically have fixed connections and weights, while DSNNs allow for dynamic changes in these parameters. This enables DSNNs to adapt to changing input distributions and learn continuously, making them more suitable for tasks that require real-time adaptation and learning.
Final Words: DSNNs represent a significant advancement in the field of neural networks, offering unparalleled flexibility and adaptability. By incorporating dynamic synaptic connections that mimic the plasticity of the human brain, DSNNs empower AI systems with the ability to learn continuously, adapt to changing environments, and deliver robust performance in a wide range of applications.