What does FBPR mean in UNCLASSIFIED
FBPR stands for Feature Based Pattern Recognition. It is a type of machine learning technique that uses features extracted from data to identify patterns and make predictions. Unlike other pattern recognition methods, FBPR focuses on the extraction of relevant features from the data, which are then used to construct a model for classification or prediction.
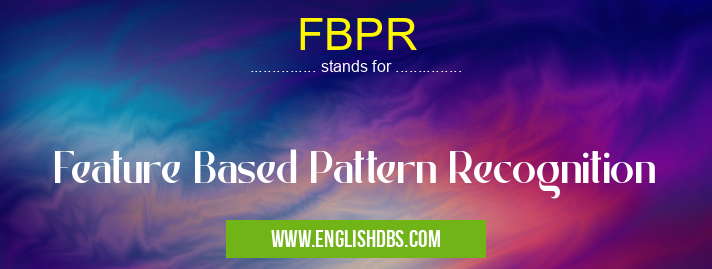
FBPR meaning in Unclassified in Miscellaneous
FBPR mostly used in an acronym Unclassified in Category Miscellaneous that means Feature Based Pattern Recognition
Shorthand: FBPR,
Full Form: Feature Based Pattern Recognition
For more information of "Feature Based Pattern Recognition", see the section below.
Types of FBPR
There are various types of FBPR techniques, including:
- Statistical FBPR: Uses statistical methods to extract features from data, such as mean, standard deviation, and correlation coefficients.
- Neural Network FBPR: Uses artificial neural networks to learn complex features from data.
- Kernel-Based FBPR: Uses kernel functions to map data into a higher-dimensional space, where features can be more easily extracted.
Applications of FBPR
FBPR has a wide range of applications in various fields, including:
- Image Recognition: Identifying objects or scenes in images
- Natural Language Processing: Understanding and classifying text
- Bioinformatics: Analyzing genetic data
- Medical Diagnosis: Detecting diseases or making prognoses
Benefits of FBPR
- Improved Accuracy: FBPR techniques can extract relevant features, leading to more accurate predictions.
- Interpretability: The features extracted by FBPR are often interpretable, providing insights into the underlying data.
- Robustness: FBPR models are generally robust to noise and outliers in the data.
Essential Questions and Answers on Feature Based Pattern Recognition in "MISCELLANEOUS»UNFILED"
What is Feature Based Pattern Recognition (FBPR)?
Feature Based Pattern Recognition (FBPR) is a technique in machine learning that identifies patterns and classifies data based on distinct features extracted from the data. It involves extracting relevant characteristics from the data, which are then used to build models that can recognize and classify patterns.
How does FBPR work?
FBPR works by first extracting features from the data. These features are then used to create feature vectors that represent each data point. The feature vectors are then used to train a classifier, which learns to identify patterns and classify data based on the extracted features.
What are the advantages of FBPR?
FBPR offers several advantages, including:
- Improved accuracy and performance in pattern recognition tasks.
- Robustness to noise and variations in the data.
- Ability to handle high-dimensional data.
- Interpretability of the extracted features.
What are the limitations of FBPR?
FBPR has some limitations, including:
- Dependence on the quality and relevance of the extracted features.
- Computational complexity for extracting features from large datasets.
- Potential for overfitting if the number of features is too high.
What are some applications of FBPR?
FBPR has a wide range of applications, such as:
- Image recognition and object detection.
- Speech recognition and natural language processing.
- Medical diagnosis and disease prediction.
- Financial fraud detection and risk assessment.
- Industrial quality control and anomaly detection.
Final Words: FBPR is a powerful pattern recognition technique that uses features extracted from data to make predictions. It has numerous applications in various fields and offers benefits such as improved accuracy, interpretability, and robustness. As machine learning continues to evolve, FBPR techniques are expected to play an increasingly important role in data analysis and decision-making.