What does VAT mean in UNCLASSIFIED
Virtual Adversarial Training (VAT) is a novel machine learning technique that uses adversarial training to improve the robustness of supervised learning models. It is designed to be used in the context of deep neural networks, and has been shown to significantly reduce overfitting in such models. In contrast to traditional adversarial training, VAT does not require manual labeling or annotating of data, making it an intriguing option for predictive analytics projects that involve large amounts of data.
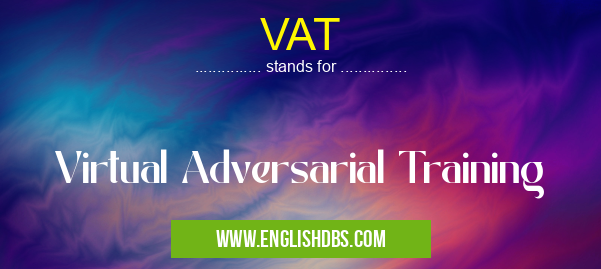
VAT meaning in Unclassified in Miscellaneous
VAT mostly used in an acronym Unclassified in Category Miscellaneous that means Virtual Adversarial Training
Shorthand: VAT,
Full Form: Virtual Adversarial Training
For more information of "Virtual Adversarial Training", see the section below.
What is VAT?
Virtual Adversarial Training (VAT) is an unsupervised machine learning technique that improves the robustness of supervised learning models by introducing virtual adversarial samples into the training process. VAT uses perturbations which are added to each sample during training, causing a model to become more resilient against small input changes and thus more robust when deployed in real-world environments. The perturbations are generated by adding modified versions of each input sample into the training process, thus creating “virtual” inputs with which a model can learn from without requiring labeled data.
Benefits of VAT
The primary benefit of using Virtual Adversarial Training (VAT) is improved robustness in supervised machine learning models without any increase in computational complexity or manual labeling requirements; this makes VAT especially suitable for predictive analytics projects involving large amounts of data. VAT also provides increased accuracy compared to other methods like Dropout and Batch Normalization; this means that models trained using VAT can generate more accurate predictions when deployed in real-world environments. Moreover, using virtual adversarial examples makes it possible to identify potential weaknesses in a model and fine-tune it accordingly before deployment – something that is not possible with manually labeled datasets alone.
Essential Questions and Answers on Virtual Adversarial Training in "MISCELLANEOUS»UNFILED"
What is Virtual Adversarial Training (VAT)?
Virtual adversarial training (VAT) is a regularization technique for neural network models that improves their robustness against perturbations in the input data. In other words, it helps networks become more resilient against slight changes in the input, like small noises or jitters which could otherwise lead to an incorrect output. The technique works by adding a virtual “adversary” to the network, which makes it difficult for the model to rely on spurious patterns and hence improves its generalization accuracy.
What are some benefits of using VAT?
Using VAT can improve deep learning networks’ overall accuracy and robustness. It can also help reduce overfitting due to noisy data points, making deep learning networks more reliable when dealing with real-world inputs. Additionally, it can be used to add robustness to datasets with noisy labels or datasets with missing labels.
How does VAT work?
VAT works by adding a “perturbation distribution” into the loss function of a neural network model. Specifically, this means adding a certain amount of noise to each input example during training so that the network starts taking into account this noise when making predictions. This makes it more difficult for the network to rely on spurious correlations and thus improves its generalization accuracy.
Is VAT limited to only neural networks?
No, although originally designed for deep learning networks, VAD has been further extended and applied to many other machine learning algorithms such as support vector machines (SVM), conditional random fields (CRF) and Naïve Bayes classifiers.
Is there any downside of using VAT?
Yes, since adding noise to inputs increases computation time significantly when compared with standard non-robust training methods, using VAT may slow down training progress by up to 50%.
Are there any applications of VAT beyond deep learning?
Yes, while initially developed for deep learning models, VAT has also been adapted for many other machine learning algorithms as well as natural language processing (NLP) tasks such as text classification and sentiment analysis.
Can I use different types of noise with VAT?
Yes, you can use different types of noise such as uniform or Gaussian with your virtual adversarial training approach depending on what type of problem you're trying to solve.
Do I need additional hardware or software requirements for running virtual adversarial training?
Generally speaking if you already have proper software installed or hardware capabilities set up then no additional requirements should be needed in order to run virtual adversarial training optimally.
Final Words:
In summary, Virtual Adversarial Training (VAT) has gained traction among researchers as an effective method for improving the robustness and accuracy of supervised neural network models without any additional computational costs or label requirements associated with traditional adversarial techniques. By introducing carefully measured perturbations into each input sample during training, VAT ensures that a model can remain resilient even under small input changes when deployed in production systems. Additionally, since virtual adversarial examples can be used to identify potential areas where a model may fail, VAT provides researchers with an efficient way to fine-tune their models before release – something which cannot be done with labeled datasets alone.
VAT also stands for: |
|
All stands for VAT |