What does WOLS mean in UNCLASSIFIED
Weighted Orthogonal Least Squares (WOLS) is a statistical method used in regression analysis to estimate the parameters of a linear model. It is an extension of Ordinary Least Squares (OLS), which is the most commonly used method for linear regression. WOLS is particularly useful when the data has heteroscedasticity, which means that the variance of the errors is not constant across observations.
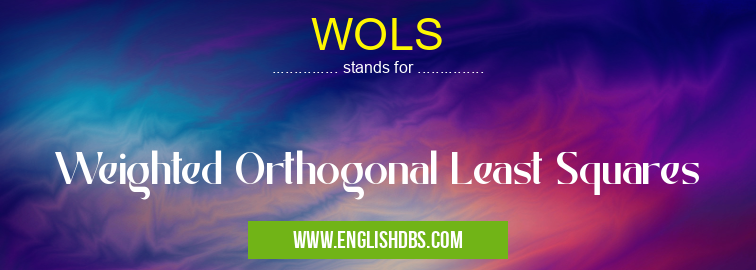
WOLS meaning in Unclassified in Miscellaneous
WOLS mostly used in an acronym Unclassified in Category Miscellaneous that means Weighted Orthogonal Least Squares
Shorthand: WOLS,
Full Form: Weighted Orthogonal Least Squares
For more information of "Weighted Orthogonal Least Squares", see the section below.
How WOLS Works
WOLS works by introducing a set of weights to the OLS regression model. These weights are inversely proportional to the variance of the errors for each observation. By weighting the observations in this way, WOLS gives more importance to observations with smaller errors and less importance to observations with larger errors. This helps to reduce the impact of heteroscedasticity on the regression results.
Advantages of WOLS
- Reduces the impact of heteroscedasticity: WOLS is specifically designed to handle heteroscedasticity, which can lead to biased and inefficient parameter estimates in OLS regression.
- More efficient parameter estimates: By giving more weight to observations with smaller errors, WOLS produces more efficient parameter estimates compared to OLS.
- Improved goodness-of-fit: WOLS can improve the goodness-of-fit of the regression model by reducing the variance of the residuals.
Essential Questions and Answers on Weighted Orthogonal Least Squares in "MISCELLANEOUS»UNFILED"
What is Weighted Orthogonal Least Squares (WOLS)?
WOLS is a statistical technique for fitting a linear regression model when the errors are not assumed to be independent and identically distributed (non-IID). It assigns weights to each data point to account for the non-IID nature of the errors, which can improve the accuracy of the model.
How does WOLS differ from ordinary least squares (OLS)?
OLS assumes that the errors are independent and identically distributed, while WOLS allows for the errors to be correlated and heteroscedastic (having unequal variances). WOLS assigns weights to each data point to compensate for these heterogeneities, which can result in a more accurate model fit.
When should WOLS be used?
WOLS is typically used when the errors in a linear regression model are known or suspected to be non-IID. This can occur in various situations, such as:
- Time series data, where observations over time are correlated
- Spatial data, where observations in close geographic proximity are correlated
- Panel data, where observations are grouped into clusters (e.g., by individuals or firms)
- Weighted regression, where some data points are considered more important than others
How is WOLS implemented?
WOLS is implemented by incorporating weights into the OLS objective function. These weights are typically determined based on the estimated covariance matrix of the errors. Various algorithms can be used to solve the weighted least squares problem, including iterative weighted least squares (IWLS) and generalized least squares (GLS).
What are the advantages of WOLS?
WOLS offers several advantages over OLS, including:
- Improved accuracy: By accounting for the non-IID nature of the errors, WOLS can produce more accurate model estimates.
- Robustness: WOLS is less sensitive to outliers and influential points than OLS, which can improve the stability of the model.
- Efficiency: WOLS can be more efficient than OLS in terms of standard errors and confidence intervals, resulting in more precise estimates.
Final Words: Weighted Orthogonal Least Squares (WOLS) is a valuable statistical method for regression analysis when the data exhibits heteroscedasticity. By weighting the observations appropriately, WOLS reduces the impact of heteroscedasticity and produces more efficient and reliable parameter estimates. It is an important tool for researchers and practitioners working with regression models.