What does PBA mean in UNCLASSIFIED
PBA (Population Based Augmentation) is a technique used in computer vision and image processing to enhance the performance of machine learning models by leveraging the knowledge derived from a diverse dataset.
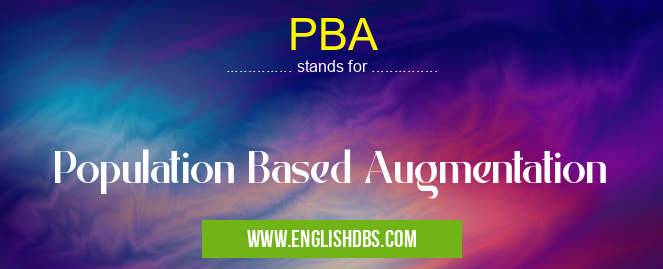
PBA meaning in Unclassified in Miscellaneous
PBA mostly used in an acronym Unclassified in Category Miscellaneous that means Population Based Augmentation
Shorthand: PBA,
Full Form: Population Based Augmentation
For more information of "Population Based Augmentation", see the section below.
Meaning of PBA
PBA involves training a machine learning model on a large, representative dataset that encompasses various domains, scenarios, and visual content. By learning from this diverse dataset, the model gains a more comprehensive understanding of the underlying visual patterns and relationships. This enriched knowledge enables the model to perform better on specific tasks, such as object detection, image classification, and segmentation, even when dealing with limited or unseen scenarios.
Advantages of PBA
- Improved generalization: PBA helps models generalize better to new and diverse data, reducing the risk of overfitting.
- Enhanced robustness: By training on a wide range of data, PBA makes models more robust to noise, occlusions, and variations in lighting and viewpoint.
- Reduced domain bias: PBA mitigates the impact of domain-specific biases that can arise when training on limited datasets, leading to more unbiased and reliable predictions.
- Increased accuracy: The comprehensive knowledge acquired through PBA enables models to achieve higher accuracy and precision in their predictions.
Applications of PBA
PBA finds applications in various fields, including:
- Object detection: Detecting and localizing objects in images and videos.
- Image classification: Categorizing images into specific classes.
- Semantic segmentation: Identifying and labeling individual pixels or regions in an image.
- Medical imaging: Analyzing medical images for disease diagnosis and treatment planning.
Essential Questions and Answers on Population Based Augmentation in "MISCELLANEOUS»UNFILED"
What is Population Based Augmentation (PBA)?
PBA is a machine learning technique that generates synthetic data from a given dataset. It leverages advanced algorithms to create new data points that are statistically similar to the original dataset, enriching it and improving model performance.
How does PBA work?
PBA starts by analyzing the original dataset to understand its statistical properties. It then employs generative models, such as Generative Adversarial Networks (GANs), to generate new data points that share similar characteristics with the original data.
What are the benefits of using PBA?
PBA offers several advantages:
- Data Augmentation: It increases the size of the dataset, providing more data for training models.
- Improved Model Performance: Synthetic data generated by PBA can enhance model accuracy and reduce overfitting.
- Preserving Privacy: PBA can create synthetic data that preserves the statistical properties of the original dataset while protecting sensitive information.
Where can PBA be applied?
PBA has a wide range of applications, including:
- Natural Language Processing: Generating synthetic text for training language models.
- Computer Vision: Creating synthetic images for object detection and classification.
- Healthcare: Augmenting medical datasets to improve diagnostic and predictive models.
Are there any limitations to PBA?
While PBA is a powerful technique, it has certain limitations:
- Computational Cost: Generating synthetic data can be computationally intensive, especially for large datasets.
- Data Quality: The quality of synthetic data depends on the accuracy of the generative model used.
- Bias: If the original dataset contains biases, PBA may also generate synthetic data with similar biases.
Final Words: PBA is a valuable technique for enhancing the performance of machine learning models in computer vision tasks. By leveraging the knowledge derived from diverse datasets, PBA helps models generalize better, improve robustness, reduce domain bias, and achieve higher accuracy. As a result, PBA has become an essential tool for developing more reliable and versatile computer vision systems.
PBA also stands for: |
|
All stands for PBA |