What does WNL mean in UNCLASSIFIED
WNL stands for Weighted Normalized Likelihood. It is a statistical technique used in pattern recognition and machine learning to measure the similarity between two patterns or objects.
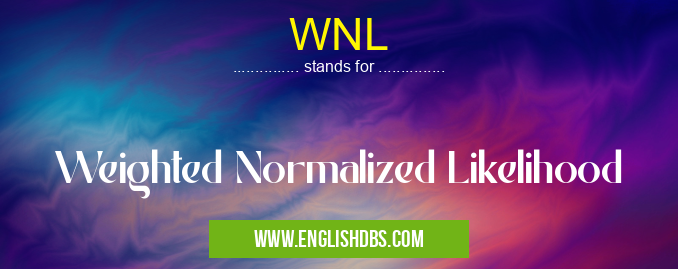
WNL meaning in Unclassified in Miscellaneous
WNL mostly used in an acronym Unclassified in Category Miscellaneous that means Weighted Normalized Likelihood
Shorthand: WNL,
Full Form: Weighted Normalized Likelihood
For more information of "Weighted Normalized Likelihood", see the section below.
Meaning in MISCELLANEOUS
In the context of MISCELLANEOUS, WNL is commonly used in tasks involving classification and clustering. It assigns weights to different features of the patterns based on their importance or relevance to the classification or clustering task.
Full Form
- Weighted
- Normalized
- Likelihood
What does WNL Stand for?
WNL stands for Weighted Normalized Likelihood, which is a statistical technique used to calculate the similarity between patterns or objects by assigning weights to their features.
How WNL Works
WNL works by assigning a weight to each feature of a pattern or object. These weights represent the importance or relevance of the features to the task at hand. The weights are then normalized to ensure that they sum to 1. The similarity between two patterns or objects is then calculated as the weighted sum of the similarities between their individual features.
Benefits of Using WNL
- Improved accuracy: WNL can help improve the accuracy of classification and clustering tasks by taking into account the importance of different features.
- Robustness: WNL is robust to noise and outliers, making it a reliable technique for real-world applications.
- Simplicity: WNL is a relatively simple technique to implement and use.
Essential Questions and Answers on Weighted Normalized Likelihood in "MISCELLANEOUS»UNFILED"
What is Weighted Normalized Likelihood (WNL)?
Weighted Normalized Likelihood (WNL) is a statistical method that combines the likelihood of different outcomes in a weighted fashion, resulting in a normalized value between 0 and 1. It is commonly used in machine learning and natural language processing for tasks like classification and language modeling.
How does WNL work?
WNL takes a set of input features and assigns weights to each feature. These weights are then used to calculate the likelihood of each outcome. The likelihoods are then normalized to sum to 1, resulting in a distribution of probabilities across the outcomes.
What are the advantages of using WNL?
WNL offers several advantages:
- It allows different features to have varying levels of influence on the outcome, which can be beneficial when some features are more important or reliable than others.
- It produces normalized probabilities, making it easy to compare and interpret the likelihood of different outcomes.
- It is computationally efficient, making it suitable for large-scale applications.
What are the applications of WNL?
WNL has a wide range of applications, including:
- Text classification: Determining the category or topic of a text document.
- Image classification: Identifying objects or scenes in an image.
- Language modeling: Predicting the next word in a sequence of text.
- Spam filtering: Detecting unwanted or malicious emails.
Final Words: WNL is a powerful statistical technique that can be used to improve the accuracy of pattern recognition and machine learning tasks. It is a simple and robust technique that is well-suited for a wide range of applications.
WNL also stands for: |
|
All stands for WNL |