What does CVAE mean in UNCLASSIFIED
Conditional Variational Auto Encoders (CVAE) are a type of generative model that combines the principles of variational autoencoders (VAEs) with conditional generation. They allow for the generation of data samples conditioned on additional information, such as class labels or other auxiliary variables.
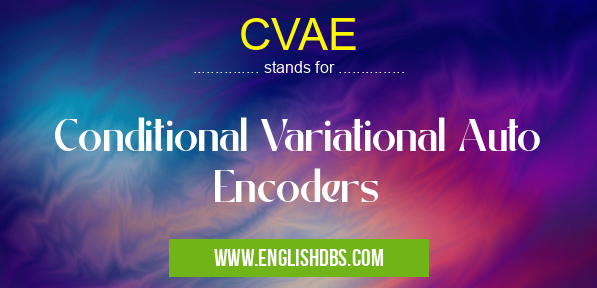
CVAE meaning in Unclassified in Miscellaneous
CVAE mostly used in an acronym Unclassified in Category Miscellaneous that means Conditional Variational Auto Encoders
Shorthand: CVAE,
Full Form: Conditional Variational Auto Encoders
For more information of "Conditional Variational Auto Encoders", see the section below.
- CVAE stands for Conditional Variational Auto Encoder.
- It is a type of generative adversarial network (GAN) that is used for unsupervised learning.
- Unconditional VAE is a generative model that learns the probability distribution of data. On the other hand, CVAE model learns a conditional probability distribution.
- CVAE is a powerful tool that can be used to generate new data, perform image recognition, and translate languages.
How does CVAE work?
- CVAE works by learning the latent distribution of data.
- The latent distribution is a probability distribution that captures the essential features of the data.
- Once the latent distribution has been learned, CVAE can be used to generate new data by sampling from the distribution.
Benefits of using CVAE
- CVAE can generate high-quality data that is similar to the original data.
- CVAE can be used for a variety of tasks, including image recognition, language translation, and drug discovery.
- CVAE is a relatively easy-to-use model that can be trained on a variety of datasets.
Applications of CVAE
- CVAE has been used to generate new images, videos, and music.
- CVAE has been used to develop new methods for image recognition and language translation.
- CVAE has been used to discover new drugs and materials.
Essential Questions and Answers on Conditional Variational Auto Encoders in "MISCELLANEOUS»UNFILED"
What are Conditional Variational Auto Encoders (CVAE)?
How do CVAEs differ from VAEs?
CVAEs extend VAEs by incorporating a conditioning variable, denoted as c. This conditioning variable provides additional information that is used to guide the generation process. The latent representation learned by the CVAE captures both the inherent structure of the data and the influence of the conditioning variable.
What are the applications of CVAEs?
CVAEs have been successfully applied in various domains, including:
- Image generation: Generating images conditioned on class labels, attributes, or other semantic information.
- Text generation: Producing text sequences conditioned on a given topic or style.
- Music generation: Composing music conditioned on genre, mood, or instrumentation.
- Data augmentation: Synthesizing new data samples to improve model performance in downstream tasks.
How are CVAEs trained?
CVAEs are trained using a combination of maximum likelihood estimation and variational inference. The loss function includes a reconstruction term that measures the similarity between the generated data and the input data, as well as a regularization term that encourages the latent representation to follow a prior distribution.
What are the advantages of using CVAEs?
CVAEs offer several advantages:
- Conditional generation: They allow for the generation of data that is consistent with the conditioning variable.
- Improved data fidelity: By incorporating conditioning information, CVAEs can generate more realistic and diverse data samples.
- Regularization: The regularization term in the loss function promotes the learning of a smooth and meaningful latent representation.
Final Words: CVAE is a powerful tool that has a wide range of applications. As the field of machine learning continues to develop, CVAE is likely to play an increasingly important role in our lives.