What does GPR mean in UNCLASSIFIED
GPR (Gaussian Process Regression) is a powerful machine learning technique used for regression and predictive modeling. It is a non-parametric Bayesian method that assumes data points follow a multivariate Gaussian distribution. GPR aims to learn the relationship between input features and outputs, enabling predictions for unseen data points.
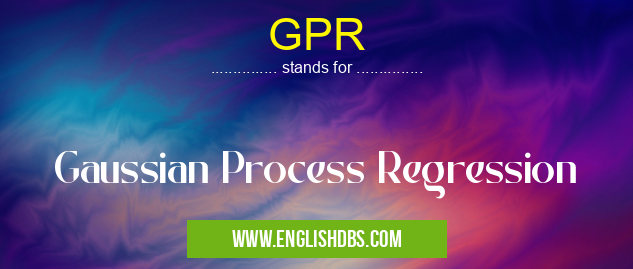
GPR meaning in Unclassified in Miscellaneous
GPR mostly used in an acronym Unclassified in Category Miscellaneous that means Gaussian Process Regression
Shorthand: GPR,
Full Form: Gaussian Process Regression
For more information of "Gaussian Process Regression", see the section below.
GPR Meaning in MISCELLANEOUS
GPR finds applications in various fields beyond machine learning, including:
- Geophysics: Subsurface exploration and characterization
- Finance: Risk assessment and forecasting
- Engineering: Structural health monitoring and damage detection
- Healthcare: Disease diagnosis and prognosis
GPR Full Form
The full form of GPR is Gaussian Process Regression.
What does GPR Stand for
GPR stands for Gaussian Process Regression, indicating that it uses Gaussian processes to model the relationship between input and output data.
Essential Questions and Answers on Gaussian Process Regression in "MISCELLANEOUS»UNFILED"
What is Gaussian Process Regression (GPR)?
GPR is a non-parametric supervised machine learning algorithm that uses Gaussian processes to model the relationship between input and output variables. It is a powerful tool for regression tasks, especially when dealing with small datasets or complex, non-linear relationships.
How does GPR work?
GPR assumes that the output variable is a realization of a Gaussian process, a stochastic process where any finite collection of random variables has a multivariate normal distribution. By defining a kernel function that measures the similarity between input points, GPR can make predictions about the output variable at new input points.
What are the advantages of GPR?
GPR offers several advantages, including:
- Flexibility: GPR can model a wide range of relationships, including non-linear and non-stationary functions.
- Uncertainty estimation: GPR provides estimates of the uncertainty associated with its predictions, which is valuable for decision making.
- Robustness: GPR is generally robust to outliers and noise in the data.
What are the disadvantages of GPR?
GPR also has some limitations:
- Computational cost: GPR can be computationally expensive for large datasets, especially when inverting the covariance matrix.
- Hyperparameter tuning: GPR requires careful tuning of its hyperparameters, which can be a challenging task.
- Interpretability: The inner workings of GPR models can be difficult to interpret due to their probabilistic nature.
What are some applications of GPR?
GPR has been successfully applied in various fields, including:
- Time series forecasting: Predicting future values of time-series data.
- Image processing: Denoising, segmentation, and image classification.
- Robotics: Modeling sensor data and predicting robot behavior.
- Material science: Characterizing and optimizing material properties.
Final Words: GPR is an advanced machine learning technique that provides a flexible and probabilistic approach to regression. Its non-parametric nature allows it to capture complex relationships in data, making it suitable for a wide range of applications in various fields. GPR's probabilistic nature enables uncertainty estimation, providing informative predictions and insights into the underlying processes.