What does AGGD mean in UNCLASSIFIED
AGGD stands for Asymmetric Generalized Gaussian Density, which is a method of statistical analysis used to describe the probability distribution of data sets with features that are not always evenly distributed. AGGD relates to a wide variety of mathematical tools and concepts, such as the Normal Distribution and the Central Limit Theorem. It can also be used to provide insights into the properties of certain probability distributions, such as estimating parameters or finding the mean and variance of a given data set. In addition, AGGD is applied in various areas including engineering, business analytics, and natural language processing (NLP) research.
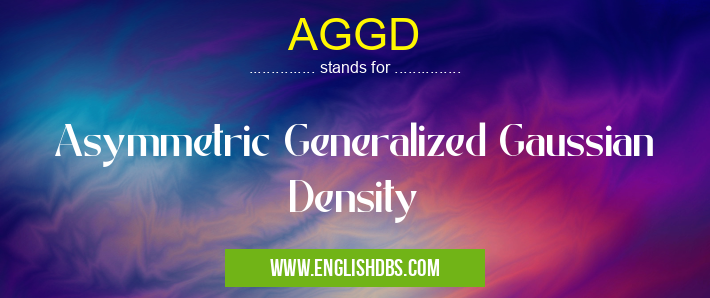
AGGD meaning in Unclassified in Miscellaneous
AGGD mostly used in an acronym Unclassified in Category Miscellaneous that means Asymmetric Generalized Gaussian Density
Shorthand: AGGD,
Full Form: Asymmetric Generalized Gaussian Density
For more information of "Asymmetric Generalized Gaussian Density", see the section below.
Definition
AGGD is a method of statistical analysis that uses the generalized Gaussian density equation to accurately model data sets with features that are not evenly distributed. This method accounts for any observed asymmetry in the data by incorporating an offset parameter into the equation which compares each point relative to its surrounding points. Furthermore, it allows for more flexibility than traditional models, like Normal and Exponential distributions by using different assumed shapes and slopes for varying distributions. As a result, AGGD provides both accurate modelling results and enhanced flexibility when handling complex datasets.
Applications
AGGD is highly useful in many areas due to its ability to produce more accurate models than traditional methods while being flexible enough to handle complex datasets. For example, in engineering applications it can be used to model various signal characteristics such as peak widths or attenuation values. In business analytics applications it can be utilized in forecasting sales or detecting market trends at a granular level; while in NLP research it can be employed to identify topics from text documents or gauge sentiment from conversations on social media platforms. The versatility of AGGD makes it a powerful tool with numerous applications across many disciplines.
Essential Questions and Answers on Asymmetric Generalized Gaussian Density in "MISCELLANEOUS»UNFILED"
What is Asymmetric Generalized Gaussian Density (AGGD)?
Asymmetric Generalized Gaussian Density (AGGD) is a type of probability density function used in statistics that can be defined using two separate parameters, which are the mean and the shape parameter. This kind of distribution is useful when you need to study data that exhibits both asymmetry and tail behaviors.
What are the applications of AGDD?
AGDD has many applications in areas such as medical imaging, signal analysis, natural language processing, machine learning and finance. It can be used to detect outliers in data sets, model non-symmetrical distributions and compare different probability distributions.
How do we calculate the mean from an AGDD dataset?
The mean of an AGDD dataset is calculated by taking the average of all data points, which can be determined by calculating the sum of values divided by the number of items. It can also be estimated from a histogram or graph representation of the data.
What is a shape parameter?
A shape parameter is a parameter used to define the nature and behavior of a probability distribution. In the case of AGDD, this parameter is important because it determines how much “tail” exists in the distribution. Higher values for this parameter indicate more tail behavior whereas lower values indicate less tail behavior.
How do I estimate an AGDD from a dataset?
To estimate an AGDD from a dataset, you need to use maximum likelihood estimation methodologies or nonlinear optimization techniques such as Expectation Maximization Algorithm. These methods will allow you to identify parameters that best approximate your dataset's underlying distribution. In addition, you may want to compare your results against known closed form formula for certain special cases to verify accuracy.
What types of datasets are suitable for fitting an AGDD model?
Any dataset which exhibits asymmetric features or show significant tail behavior is well suited for fitting with an AGDD model. Examples include datasets with heavy-tailed distributions such as financial time series, biological signals or natural language processing documents which contain words that appears rarely but often over longer windows of text than usual.
How can I evaluate my results after fitting an AGGGD model?
After fitting an AGGD model on your dataset, you can evaluate its results using various statistical measures such as Mean Squared Error (MSE), Root Mean Squared Deviation (RMSD) or Kolmogorov-Smirnov test statistic (KS). These evaluation metrics will help you assess how closely your fitted model matches the target distribution represented by your data set.
Is there any software available for computing AGGD models?
Yes, there are several software packages available which include functions for estimating AGGD models including R package gfit1d_asymmetric_gaussian(), SAS proc global fit(), SCILAB fitagggd() and MATLAB agggd(). Additionally there are many open source libraries specifically designed for computing AGGD models such as GPML Toolbox for Matlab, Scipy Distributions Library and Scikit Learn Library for Python.
Final Words:
In conclusion, AGGD stands for Asymmetric Generalized Gaussian Density which is an advanced form of statistical analysis that utilizes an offset parameter to account for any observed asymmetry within data sets with unevenly distributed features, thereby providing improved accuracy compared traditional models while still being flexible enough to handle complex datasets effectively. Its versatility has resulted in AGGD becoming increasingly popular among numerous disciplines ranging from engineering and business analytics all the way through Natural Language Processing (NLP) research; proving itself as a highly useful tool worth considering when addressing modern day problems requiring accurate modelling results combined with enhanced flexibility.