What does BODF mean in UNCLASSIFIED
Bag of Deep Features (BODF) is a deep learning method developed by researchers at IBM Research Labs. It is a powerful tool to transform text data into numerical features that can be used by machine learning models for various tasks, such as document classification, text analysis, and sentiment analysis. The BODF algorithm uses unsupervised learning in order to automatically identify significant topics within the text and generate meaningful numeric representations of them. This process results in a bag of deeply learned features which may then be fed into downstream applications such as machine learning models or natural language processing systems.
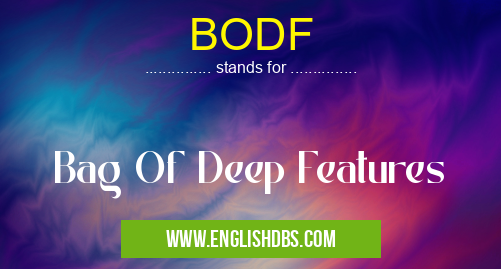
BODF meaning in Unclassified in Miscellaneous
BODF mostly used in an acronym Unclassified in Category Miscellaneous that means Bag Of Deep Features
Shorthand: BODF,
Full Form: Bag Of Deep Features
For more information of "Bag Of Deep Features", see the section below.
Essential Questions and Answers on Bag Of Deep Features in "MISCELLANEOUS»UNFILED"
What is Bag of Deep Features (BODF)?
Bag of Deep Features (BODF) is an unsupervised learning technique used to extract meaningful features from images. It uses convolutional neural networks to learn visual patterns from large collections of images, resulting in a set of feature vectors for each image. The feature vector is then used as input to a classification algorithm to classify the image into one or more categories.
What are the benefits of using BODF?
There are several benefits of using Bag Of Deep Features (BODF). Firstly, it enables the efficient extraction of deep features which can be used for image classification tasks such as object recognition and scene understanding. Secondly, it offers improved accuracy and robustness over traditional methods for feature extraction. Finally, it provides an unsupervised approach that can be applied to datasets with no human annotation available.
How does BODF work?
BODF works by first using a convolutional neural network (CNN) model to learn good representations from a large collection of natural images. This model produces a feature vector from each image, which can be used as input to a classification algorithm for categorizing the images into one or more classes.
What types of applications can BODF be used for?
BODF has been applied successfully in various computer vision tasks such as object recognition, scene understanding and image retrieval. It has also been used for autonomous robotics navigation systems and various other industrial automation tasks such as automated surveillance and monitoring systems.
Is BODF easy to implement?
Yes, Bag Of Deep Features (BODF) is relatively easy to implement since it requires only standard components like convolutional neural networks models and open-source machine learning algorithms such as Support Vector Machines (SVM). Furthermore, most existing implementations are quite modular so they allow users to easily introduce new components or extend existing ones without major changes in the codebase itself.
How much data is required by BODF?
The amount of data required by Bag Of Deep Features (BODF) depends on the complexity of the task at hand but typically a large dataset consisting of tens of thousands or even millions of images is necessary in order to achieve good results with this technique. Additionally, the quality and diversity of the dataset also have impact on its performance so collecting high-quality samples from different contexts should be considered when deploying this method for any given task.
Is BODF language independent?
Yes, Bag Of Deep Features (BODF) is language independent since it deals solely with extracted features from images and not actual textual content associated with them. This makes it possible to apply this technique across multiple languages without requiring additional modifications or changes in its implementation itself.
Final Words:
Bag Of Deep Features (BODF) is an unsupervised deep learning technique developed by researchers at IBM Research Labs for extracting semantically meaningful numerical representations from text-based datasets with the aim of improving downstream machine learning applications such as document classification, text analysis, and sentiment analysis tasks. Despite its complexity, BODF offers an effective solution for automatically extracting high-level features from text-based datasets without requiring manual feature engineering efforts beforehand.