What does EVD mean in UNCLASSIFIED
Eigen Value Decomposition (EVD) is a process of decomposing a square matrix or linear operator into its constituent eigenvalues and vectors. It is a useful tool for understanding the behavior of complex systems and analyzing their underlying structure. Eigenvalues are scalar values that can be used to measure the strength of relationships between different elements of a system, while Eigenvectors represent directions in which those relationships point. EVD allows for efficient representation of complex systems and can provide insight into how those systems behave over time.
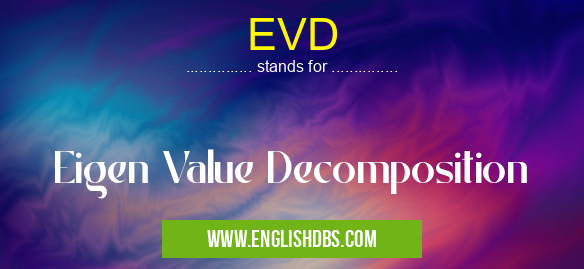
EVD meaning in Unclassified in Miscellaneous
EVD mostly used in an acronym Unclassified in Category Miscellaneous that means Eigen Value Decomposition
Shorthand: EVD,
Full Form: Eigen Value Decomposition
For more information of "Eigen Value Decomposition", see the section below.
Explanation
Eigen Value Decomposition is used in many areas within mathematics and applied mathematics. It is especially useful in dynamical systems, where it provides insight into the behavior of those systems by breaking them down into their individual components. On one hand, EVD can provide information about the stability, controllability, or observability of a system by identifying significant eigenspaces associated with the dynamics; on the other hand, it can also be used to estimate global dynamic parameters such as maximum-entropy states, Lyapunov exponents, and average entropy for chaotic systems. Furthermore, EVD finds applications in control theory for determining optimal control strategies that maximize performance or reduce cost; linear algebraic techniques such as principal component analysis; optimization problems involving matrix decompositions; and singular value decomposition-based approximation methods.
Essential Questions and Answers on Eigen Value Decomposition in "MISCELLANEOUS»UNFILED"
What is Eigen Value Decomposition (EVD)?
Eigen Value Decomposition (EVD) is a mathematical technique used to decompose a matrix into its constituent parts. It is most commonly used in linear algebra and data analysis, where it can be used to identify patterns within large datasets. EVD works by decomposing the data into its eigenvectors and then calculating the corresponding eigenvalues. This allows the dataset to be studied at a more granular level.
How does EVD work?
EVD works by decomposing a given matrix into its eigenvectors and associated eigenvalues. The eigenvectors are calculated first, which are then used to calculate the corresponding eigenvalues. The resulting decomposition provides insight into the structure of the original matrix, as well as providing valuable information about the data contained within it.
What types of data can be analyzed using EVD?
EVD can be applied to any type of numerical or categorical dataset, including both text-based and image-based datasets. It can also be used on datasets containing both continuous and categorical variables, making it an ideal tool for analyzing complex datasets.
What are the benefits of using EVD?
There are numerous benefits to using EVD for analyzing data sets. First, it simplifies complex datasets by allowing us to break them down into their constituent parts in order to better understand their structure and content. Additionally, this technique enables us to perform detailed analyses on subsets of our original dataset without having to recalculate or reanalyze any information from scratch. Finally, EVD provides powerful insights that may not have been readily apparent before performing the decomposition process on our dataset.
Is there anything special that needs to be done before starting an EVD?
Generally speaking no prior preparation is required before performing an Eigen Value Decomposition (EVD). However, depending on the type of data being analyzed some preprocessing steps may need taken in order to properly prepare your dataset for successful decomposition. If you’re unsure if your current setup will adequately support an EVD it’s always best practice top consult with someone who has experience working with similar datasets beforehand.
Are there any disadvantages associated with using Eigen Value Decomposition?
While there are many advantages associated with employing Eigen Value Decomposition on one’s dataset, there may also be some potential drawbacks depending upon one’s specific use case scenario. Firstly, while Eigen values provide useful insights they can only provide so much about a given dataset; often times additional exploration must still take place outside of EVD in order for further insights about one’s data set to be revealed. Additionally, due to how computationally intensive this technique is it may not always prove feasible under certain resource constraints.
Final Words:
In conclusion, Eigen Value Decomposition is an important tool for understanding complex systems and analyzing their structure more effectively. Its application has broad implications across various scientific fields including physics, engineering, computer science and finance. Moreover, its flexibility allows it to provide insights into various types of linear dynamical systems as well as optimization problems involving matrix decompositions. Therefore this technique is invaluable for researchers seeking to gain deeper knowledge about these complicated subjects.
EVD also stands for: |
|
All stands for EVD |