What does EDBN mean in UNCLASSIFIED
Extended Deep Belief Network (EDBN) is a generative artificial neural network which utilizes unsupervised learning to discover and model the complex relationships existing within data. It was developed by Geoffrey Hinton, Ruslan Salakhutdinov and Yoshua Bengio as an extension of the traditional deep belief network. EDBN has the capability to produce multiple layers of connected neurons which allow it to detect patterns in data beyond what typical networks are capable of. Additionally, EDBN also provides improved performance and better accuracy when compared to regular deep belief networks.
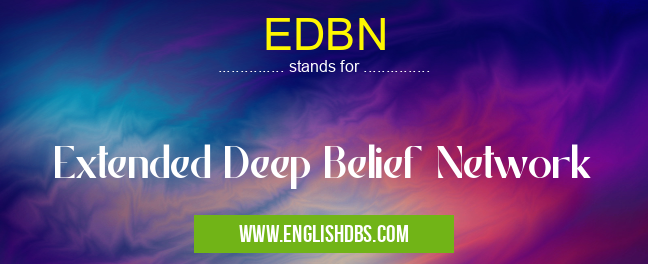
EDBN meaning in Unclassified in Miscellaneous
EDBN mostly used in an acronym Unclassified in Category Miscellaneous that means Extended Deep Belief Network
Shorthand: EDBN,
Full Form: Extended Deep Belief Network
For more information of "Extended Deep Belief Network", see the section below.
Essential Questions and Answers on Extended Deep Belief Network in "MISCELLANEOUS»UNFILED"
What is an Extended Deep Belief Network?
An extended deep belief network (EDBN) is a type of artificial neural network that combines several layers of deep belief networks in order to create a better model for tasks such as classification and regression. It utilizes both supervised and unsupervised learning mechanisms. EDBNs are usually used for large-scale problems where the data set has high dimensional complexity, such as image or video recognition.
How does an EDBN differ from a regular deep belief network?
A regular deep belief network utilizes only unsupervised learning, while an EDBN uses both supervised and unsupervised techniques. The use of supervised learning reduces over-fitting, allowing the model to generalize better on unseen data points. Furthermore, the number of layers in an EDBN can be modified according to the complexity of the task in question.
How do EDBNs learn?
EDBNs learn by constructing representations from lower level features. Each layer of the network is trained on data with labels associated with it to capture patterns in the data. The next layer then builds upon these representations to learn more complex structures and features that help identify correlations between variables. This process allows EDBNs to learn faster than other methods on large datasets with complex relationships between variables.
What types of tasks can be solved using an EDBN?
EDBNs can be used for various tasks including classification, clustering, feature extraction, pattern recognition, anomaly detection, reinforcement learning, and time series prediction. They are particularly useful when dealing with high dimensional problems that involve complex patterns or require multiple levels of abstraction.
What are some advantages of using an EDBN over a regular DNN?
Some advantages include faster training times due to fewer layers in comparison to DNNs, improved accuracy due to understanding of patterns through multiple levels of abstraction, greater flexibility in terms of adjusting hyperparameters according to specific tasks,,and better generalization performance due to less over-fitting.
Are there any disadvantages associated with using an Extended Deep Belief Network?
One potential disadvantage is that its structure can make it difficult for practitioners unfamiliar with this type of architecture to understand how it functions and what it is capable of achieving. Additionally, due to its design incorporating both supervised and unsupervised learning components which each perform separate computations on different subsets of data; they may take longer to train than single-layer models.
Is there any way to reduce training times when working with an Extended Deep Belief Network?
Yes! One way to reduce training time is by utilizing GPU acceleration which allows many processes within each model layer can be computed simultaneously instead only computing them one at a time serially by CPU’s. Furthermore specialized libraries such as Google’s TensorFlow provide built-in support for taking advantage GPUs during training.
Final Words:
In conclusion, Extended Deep Belief Networks are a powerful artificial intelligence tool that can be used for many applications where accurate results from complex datasets are needed. Its combination of Restricted Boltzmann Machines alongside various generative models allows it to discover correlations in larger volumes of data than previously achievable with simple DBN’s. Furthermore, its ability for real-time processing makes it suitable for tasks that require high speed learning capabilities in order for them to be completed quickly and reliably. All these qualities make Extended Deep Belief Networks both versatile and applicable for many potential use cases across multiple industries.