What does DPC mean in UNCLASSIFIED
In the realm of Miscellaneous terminology, DPC stands for Density of Points Clustering. It is a significant concept in data mining and cluster analysis, providing a means to determine the density of data points within a specific region.
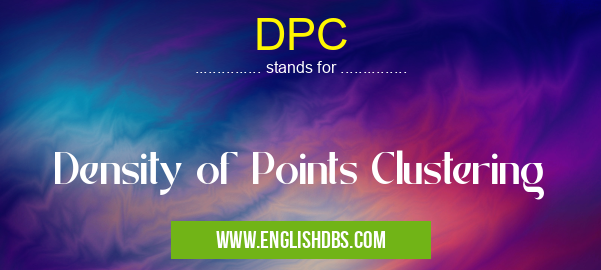
DPC meaning in Unclassified in Miscellaneous
DPC mostly used in an acronym Unclassified in Category Miscellaneous that means Density of Points Clustering
Shorthand: DPC,
Full Form: Density of Points Clustering
For more information of "Density of Points Clustering", see the section below.
Definition of DPC
DPC measures the number of data points that exist in a given space, providing an indication of the concentration of data within that area. It is calculated by dividing the number of points within a cluster by the volume of the cluster's bounding box. A higher DPC value signifies a denser cluster, while a lower value indicates a more dispersed distribution of points.
Applications of DPC
DPC finds numerous applications across various fields:
- Data Mining: Identifying clusters of data points in high-dimensional datasets, which can reveal hidden patterns and structures.
- Image Processing: Segmenting images into different regions based on the density of pixels, allowing for object recognition and feature extraction.
- Geographic Information Systems (GIS): Analyzing the spatial distribution of data points, such as population density or resource allocation.
Advantages of Using DPC
- Simplicity: It is a straightforward and easy-to-calculate metric.
- Interpretability: DPC provides a clear understanding of the concentration of data points within a cluster.
- Robustness: It is relatively insensitive to noise and outliers in the data.
Essential Questions and Answers on Density of Points Clustering in "MISCELLANEOUS»UNFILED"
What is Density of Points Clustering (DPC)?
Density of Points Clustering (DPC) is a clustering technique used in machine learning and data analysis to identify clusters of points in a dataset based on their density. It assigns each point a density value based on the number of points in its neighborhood and then groups points with similar density values into clusters.
How does DPC work?
DPC works by calculating the density of each point in a dataset. This is typically done by counting the number of points within a specified radius of each point. Points with a higher density are more likely to belong to the same cluster. The density values are then used to assign points to clusters. Points with similar density values are grouped together, while points with different density values are assigned to different clusters.
What are the advantages of using DPC?
DPC has several advantages, including:
- It is able to identify clusters of arbitrary shape and size.
- It is relatively insensitive to noise and outliers in the data.
- It is computationally efficient, making it suitable for large datasets.
What are the limitations of using DPC?
DPC also has some limitations, such as:
- It can be sensitive to the choice of radius used to calculate density.
- It can be difficult to determine the optimal number of clusters in a dataset.
- It can be challenging to handle datasets with a high degree of overlap between clusters.
What are some applications of DPC?
DPC has a wide range of applications, including:
- Image segmentation
- Object detection
- Anomaly detection
- Customer segmentation
- Social network analysis
Final Words: DPC is a useful metric for assessing the density of data points within a cluster, with applications in various domains. Its simplicity, interpretability, and robustness make it a valuable tool for data analysis and exploration. By understanding the concept of DPC, practitioners can gain valuable insights into the underlying structure and distribution of data.
DPC also stands for: |
|
All stands for DPC |