What does QC mean in UNCLASSIFIED
In the context of MISCELLANEOUS, QC refers to the use of Quadratic Classification for various tasks, such as:
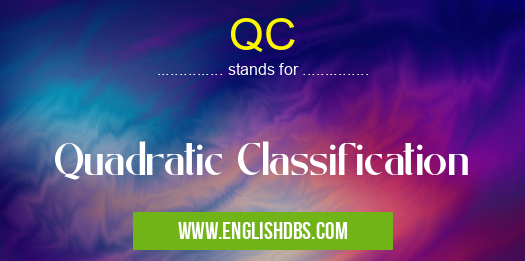
QC meaning in Unclassified in Miscellaneous
QC mostly used in an acronym Unclassified in Category Miscellaneous that means Quadratic Classification
Shorthand: QC,
Full Form: Quadratic Classification
For more information of "Quadratic Classification", see the section below.
QC Meaning in MISCELLANEOUS
- Image classification: Identifying objects in images
- Natural language processing: Categorizing text into different topics
- Medical diagnosis: Classifying patients based on their symptoms
QC Full Form
- Quadratic Classification
What Does QC Stand For?
QC stands for Quadratic Classification, a method that:
- Uses quadratic equations to model the relationship between data points and class labels
- Allows for complex decision boundaries to accurately separate different classes
Essential Questions and Answers on Quadratic Classification in "MISCELLANEOUS»UNFILED"
What is Quadratic Classification (QC)?
QC is a supervised machine learning algorithm used for binary classification problems. It creates a quadratic decision boundary, which is a curved surface in the feature space, to separate the two classes. QC models complex relationships between features and the target variable.
How does QC differ from other classification algorithms?
Unlike linear classifiers (e.g., logistic regression), which use a straight line as the decision boundary, QC allows for non-linear relationships. It captures quadratic interactions and curvature, providing greater flexibility in modeling complex data patterns.
What are the advantages of using QC?
QC offers several advantages:
- Non-linearity: Handles complex relationships between features and the target variable.
- Flexibility: Captures quadratic interactions and curvature, allowing for more accurate modeling.
- Robustness: Less prone to overfitting compared to other non-linear classifiers.
When should I use QC?
QC is suitable for situations where:
- The data exhibits non-linear relationships between features and the target variable.
- The decision boundary is expected to be curved.
- Other linear classifiers fail to capture the complexity of the data.
What are the limitations of QC?
QC has some limitations:
- Computational complexity: The training process can be computationally intensive, especially for large datasets.
- Interpretability: The quadratic decision boundary can be difficult to interpret and visualize.
- Regularization: Requires careful regularization to prevent overfitting.
How can I choose the appropriate kernel for QC?
The choice of kernel is crucial in QC. Common kernels include:
- Gaussian kernel: Suitable for data with non-linear relationships and high dimensionality.
- Polynomial kernel: Captures polynomial interactions of features.
- Sigmoid kernel: Used in support vector machines for non-linear classification.
Final Words: QC, or Quadratic Classification, is a powerful tool for classifying data points into different categories. It is widely used in various fields, including image processing, natural language processing, and medical diagnosis. By understanding its principles and applications, you can leverage QC to extract valuable insights from your data.
QC also stands for: |
|
All stands for QC |