What does LQS mean in UNCLASSIFIED
Least Quantile of Squares (LQS) is a statistical technique used to estimate the quantiles of a distribution, particularly the median, using a least squares approach. It is a generalization of the least squares regression model, where the goal is to minimize the sum of squared errors between the observed data and the estimated quantiles.
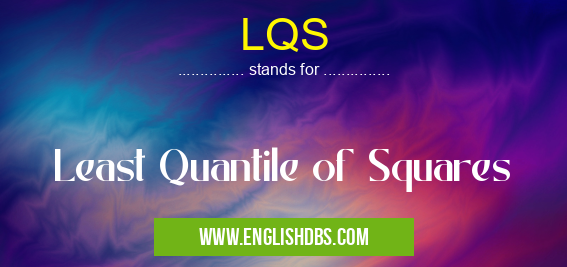
LQS meaning in Unclassified in Miscellaneous
LQS mostly used in an acronym Unclassified in Category Miscellaneous that means Least Quantile of Squares
Shorthand: LQS,
Full Form: Least Quantile of Squares
For more information of "Least Quantile of Squares", see the section below.
LQS Meaning
LQS stands for Least Quantile of Squares. It is a method for finding the quantiles of a distribution by minimizing the sum of squared errors between the observed data and the estimated quantiles.
LQS Full Form
Least Quantile of Squares
What does LQS Stand for?
LQS stands for Least Quantile of Squares. It is a statistical technique used to estimate the quantiles of a distribution, particularly the median, using a least squares approach.
Advantages of LQS
- Robustness: LQS is relatively robust to outliers and extreme values, making it suitable for analyzing data with non-normal distributions.
- Efficiency: LQS can provide efficient estimates of quantiles, especially for large datasets.
- Interpretability: LQS estimates have a clear geometrical interpretation and can be easily understood.
- Flexibility: LQS can incorporate additional information or constraints into the estimation process, making it suitable for a variety of applications.
Applications of LQS
- Risk assessment: Estimating the quantiles of risk factors, such as financial losses or insurance claims.
- Data analysis: Finding the median, quartiles, or other quantiles of large datasets to summarize their distribution.
- Time series analysis: Estimating the quantiles of time series data to identify patterns and trends.
- Regression analysis: Incorporating quantile regression into regression models to account for non-linear relationships between variables.
Essential Questions and Answers on Least Quantile of Squares in "MISCELLANEOUS»UNFILED"
What is the Least Quantile of Squares (LQS)?
LQS is a statistical technique used for robust regression analysis. It is designed to minimize the sum of the weighted absolute residuals, making it less sensitive to outliers compared to traditional least squares regression.
How does LQS differ from traditional least squares regression?
Traditional least squares regression minimizes the sum of the squared residuals, which gives more weight to large errors. In contrast, LQS uses the median of the absolute residuals, giving less weight to outliers and making it more robust.
When should I use LQS?
LQS is particularly useful when dealing with data that contains outliers or heavy-tailed distributions. It is also helpful when the errors are not normally distributed, as it does not assume a specific error distribution.
What are the advantages of using LQS?
LQS offers several advantages:
- Robustness against outliers and heavy-tailed distributions
- Less sensitive to deviations from normality
- Less computationally intensive than other robust regression methods
What are the limitations of LQS?
While LQS is a powerful technique, it also has some limitations:
- May not be as efficient as least squares regression in the absence of outliers
- Can be sensitive to the choice of quantile used
- May not perform well with small sample sizes
Final Words: Least Quantile of Squares (LQS) is a valuable statistical technique for estimating quantiles of distributions, particularly the median. Its robustness, efficiency, interpretability, and flexibility make it a useful tool for various applications in risk assessment, data analysis, time series analysis, and regression analysis.
LQS also stands for: |
|
All stands for LQS |