What does SFFS mean in UNCLASSIFIED
SFFS is an abbreviation for Sequential Forward Floating Selection, which is a machine learning algorithm used to determine the best combination of features from a given set of data. It is commonly used in predictive modelling and is especially useful for datasets with multiple input variables. This technique helps identify which features add the most value in predicting a target variable by calculating values such as coefficient of determination, mean squared error and other performance metrics.
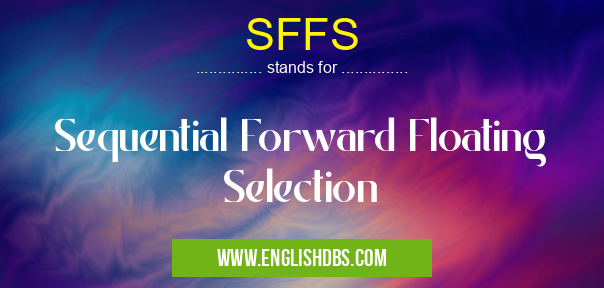
SFFS meaning in Unclassified in Miscellaneous
SFFS mostly used in an acronym Unclassified in Category Miscellaneous that means Sequential Forward Floating Selection
Shorthand: SFFS,
Full Form: Sequential Forward Floating Selection
For more information of "Sequential Forward Floating Selection", see the section below.
Essential Questions and Answers on Sequential Forward Floating Selection in "MISCELLANEOUS»UNFILED"
What does SFFS stand for?
SFFS stands for Sequential Forward Floating Selection.
How does SFFS work?
SFFS works by repeatedly selecting, testing and evaluating the effectiveness of each feature in predicting the desired target variable. It creates a model using the selected features, which can then be evaluated against different criteria to find the optimal combination that provides the best results.
What are some common uses for SFFS?
SFFS is commonly used in predictive modelling applications such as regression and classification problems. It can also be used to optimize parameters in neural networks or deep learning models.
Is it possible to use SFFS with unsupervised data?
Yes, although SFFS is primarily used with supervised datasets that have defined target variables, it can also be used with unsupervised datasets. However, its ability to effectively identify optimal features may be limited due to not having available labels or targets to evaluate against.
Does SFFS provide any additional benefits beyond feature selection?
Yes, besides providing an efficient way to identify relevant features from a dataset, it also helps enhance interpretability by allowing deeper insights into how individual variables influence predictions or outcomes of machine learning models.
Final Words:
In conclusion, Sequential Forward Floating Selection (SFFs) is an important tool in predictive analytics that helps optimize performance through feature selection while also enhancing interpretability and understanding around how individual variables impact outcomes.