What does ABIC mean in UNCLASSIFIED
ABIC (Akaike Bayesian Information Criterion) is a statistical measure used for model selection, particularly in Bayesian inference. It combines the likelihood of a model with a penalty term that discourages overfitting, making it useful in situations where there are multiple models to choose from.
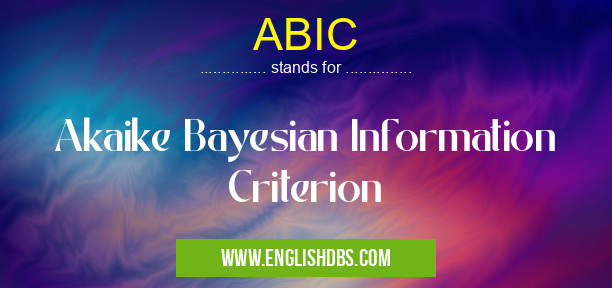
ABIC meaning in Unclassified in Miscellaneous
ABIC mostly used in an acronym Unclassified in Category Miscellaneous that means Akaike Bayesian Information Criterion
Shorthand: ABIC,
Full Form: Akaike Bayesian Information Criterion
For more information of "Akaike Bayesian Information Criterion", see the section below.
Meaning of ABIC
ABIC is based on the Akaike Information Criterion (AIC), which penalizes models for their complexity. The Bayesian extension of AIC adds a term that accounts for the uncertainty in the model parameters. This additional term encourages models that are not only well-supported by the data but also have relatively few parameters, reducing the risk of overfitting.
Calculation of ABIC
The formula for ABIC is given by:
ABIC = -2 * log(p(y|x, θ)) + 2 * K + log(n)
where:
- p(y|x, θ) is the likelihood of the data y given the parameters θ and model x.
- K is the number of parameters in the model.
- n is the sample size.
Interpretation of ABIC
Lower ABIC values indicate better models. The difference in ABIC values between two models can be used to assess the relative support for each model. A difference of 2 or more in ABIC values is considered to be significant, providing moderate evidence in favor of the model with the lower ABIC.
Advantages of ABIC
- Penalizes overfitting by discouraging complex models.
- Accounts for uncertainty in model parameters.
- Can be used to compare non-nested models.
Applications of ABIC
ABIC is widely used in various fields, including:
- Model selection in Bayesian statistics.
- Hypothesis testing and variable selection.
- Machine learning and artificial intelligence.
Essential Questions and Answers on Akaike Bayesian Information Criterion in "MISCELLANEOUS»UNFILED"
What is the Akaike Bayesian Information Criterion (ABIC)?
The Akaike Bayesian Information Criterion (ABIC) is a statistical measure used to select among competing models for a given set of data. It is an extension of the Akaike Information Criterion (AIC) that incorporates Bayesian principles.
How is ABIC calculated? A: ABIC is calculated using the following formul
ABIC is calculated using the following formula:
ABIC = AIC + 2k
where:
- AIC is the Akaike Information Criterion
- k is the number of estimated parameters in the model
What is the purpose of ABIC?
The purpose of ABIC is to provide a way to compare models that have different numbers of parameters and different levels of complexity. The model with the lowest ABIC value is considered the best model.
How is ABIC different from AIC?
ABIC differs from AIC in that it incorporates Bayesian principles. This means that ABIC takes into account the uncertainty in the estimated parameters and the prior probability of the model.
When should ABIC be used?
ABIC should be used when comparing models that have different numbers of parameters and different levels of complexity. It is particularly useful when the sample size is small or when there is a large number of parameters in the model.
Final Words: ABIC is a valuable tool for model selection in Bayesian inference. It helps to identify models that are both well-supported by the data and have relatively few parameters, reducing the risk of overfitting. By considering both the likelihood and the complexity of a model, ABIC provides a robust and reliable measure for comparing and selecting among competing models.
ABIC also stands for: |
|
All stands for ABIC |