What does ANNE mean in MUSIC
Artificial Neural Network Ensemble (ANNE) is a powerful machine learning technique that utilizes an ensemble of artificial neural networks (ANNs) to enhance the overall performance and accuracy of a predictive model.
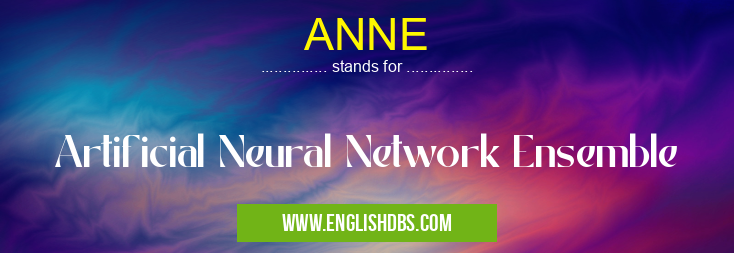
ANNE meaning in Music in Community
ANNE mostly used in an acronym Music in Category Community that means Artificial Neural Network Ensemble
Shorthand: ANNE,
Full Form: Artificial Neural Network Ensemble
For more information of "Artificial Neural Network Ensemble", see the section below.
What is ANNE?
ANNE is a combination of multiple ANNs, typically trained on different subsets of data or using different training algorithms. Each individual ANN within the ensemble makes predictions independently, and their outputs are then combined or averaged to produce a final prediction. By leveraging the collective knowledge and strengths of multiple ANNs, ANNE aims to mitigate individual weaknesses, improve generalization ability, and achieve more robust and reliable results.
Benefits of ANNE
ANNE offers several advantages over single ANNs:
- Increased Accuracy: Combining multiple ANNs reduces prediction errors and enhances the overall accuracy of the model.
- Reduced Overfitting: By training on different data subsets, ANNE helps prevent individual ANNs from overfitting to specific features, resulting in a more generalized model.
- Enhanced Robustness: The diversity of ANNs within the ensemble makes ANNE less susceptible to noise or outliers in the data, leading to more stable predictions.
- Parallel Processing: The independent nature of ANNs within the ensemble allows for parallel processing, reducing training and prediction times.
Applications of ANNE
ANNE has found applications in various domains, including:
- Image classification and recognition
- Natural language processing
- Time series forecasting
- Medical diagnosis and prognosis
Essential Questions and Answers on Artificial Neural Network Ensemble in "COMMUNITY»MUSIC"
What is ANNE?
ANNE stands for Artificial Neural Network Ensemble, which is a powerful machine learning technique that combines multiple neural networks to enhance performance.
How does ANNE work?
ANNE involves training an ensemble of neural networks, each with its own architecture and parameters. These networks are trained on the same dataset and then combined to make predictions or solve problems. By leveraging the collective strengths of individual networks, ANNE aims to improve accuracy, robustness, and generalization capabilities.
What are the benefits of using ANNE?
ANNE offers several advantages, including:
- Enhanced accuracy and reliability: By combining multiple neural networks, ANNE reduces the risk of overfitting and improves the overall accuracy of predictions.
- Robustness: The ensemble approach makes ANNE less susceptible to noise and outliers in the data, leading to more robust and stable performance.
- Generalization capabilities: ANNE can generalize better to unseen data compared to single neural networks, as it incorporates diverse perspectives and reduces the impact of specific network biases.
Where can ANNE be applied?
ANNE has a wide range of applications, including:
- Image and object recognition
- Natural language processing
- Speech recognition
- Predictive modeling
- Financial forecasting
What are the limitations of ANNE?
While ANNE offers many benefits, it also has some limitations:
- Computational complexity: Training and deploying an ensemble of neural networks can be computationally demanding, especially for large datasets.
- Hyperparameter tuning: ANNE involves tuning numerous hyperparameters, which can be a time-consuming and challenging task.
- Interpretability: Understanding the predictions made by ANNE can be challenging due to the complex interactions within the ensemble.
Final Words: ANNE is an effective machine learning technique that harnesses the power of multiple ANNs to achieve superior performance and accuracy. By combining the strengths of individual models, ANNE reduces overfitting, enhances generalization, and delivers more robust and reliable predictions, making it a valuable tool for a wide range of data analysis and predictive modeling tasks.