What does LWLS mean in UNCLASSIFIED
LWLS stands for Linear Weighted Least Squares, a robust statistical method used to estimate unknown parameters in a linear regression model when the errors are heteroskedastic, meaning the variance of the errors differs across observations.
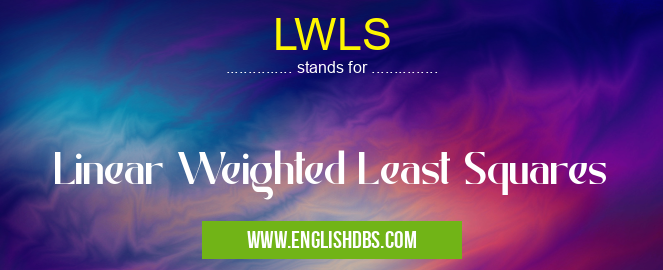
LWLS meaning in Unclassified in Miscellaneous
LWLS mostly used in an acronym Unclassified in Category Miscellaneous that means Linear Weighted Least Squares
Shorthand: LWLS,
Full Form: Linear Weighted Least Squares
For more information of "Linear Weighted Least Squares", see the section below.
LWLS Process
In LWLS, each data point is assigned a weight based on its estimated variance. Points with higher variance (more uncertain) receive lower weights, while points with lower variance (more certain) receive higher weights. This weighting scheme downplays the influence of less reliable data points, allowing for more accurate parameter estimation.
Advantages of LWLS
- Robustness: LWLS is less sensitive to outliers and influential data points compared to ordinary least squares (OLS).
- Efficiency: When the error variance is known or can be estimated accurately, LWLS can provide more efficient parameter estimates than OLS.
Applications
LWLS finds applications in various fields, including:
- Finance: Estimating financial parameters, such as stock returns and risk premiums.
- Econometrics: Estimating economic relationships where heteroskedasticity is present.
- Environmental Science: Analyzing environmental data, where measurements may have varying levels of uncertainty.
Essential Questions and Answers on Linear Weighted Least Squares in "MISCELLANEOUS»UNFILED"
What is Linear Weighted Least Squares (LWLS)?
LWLS is a statistical method used in regression analysis to address the issue of heteroscedasticity, where the variance of the errors is not constant across observations. It assigns weights to each data point, with the weights inversely proportional to the variance of the errors. By doing so, it ensures that data points with higher variance have less influence on the regression model, resulting in more accurate and reliable parameter estimates.
When is LWLS appropriate to use?
LWLS is suitable when there is evidence of heteroscedasticity in the data. This can be detected through diagnostic tests, such as the Breusch-Pagan test or the White test. If the test results indicate significant heteroscedasticity, using LWLS can improve the accuracy of the regression model.
How are the weights determined in LWLS?
The weights in LWLS are calculated as the inverse of the variance of the errors. This variance can be estimated using various methods, such as the Cochrane-Orcutt procedure or the estimated generalized least squares (EGLS) method.
What are the benefits of using LWLS?
LWLS has several benefits:
- It reduces the bias caused by heteroscedasticity, leading to more accurate parameter estimates.
- It improves the efficiency of the regression model by giving more weight to data points with lower variance.
- It can stabilize the variance of the residuals, making the model more robust.
Are there any limitations to LWLS?
While LWLS is a powerful technique, it has some limitations:
- It requires an accurate estimate of the variance of the errors, which can be challenging in practice.
- It can be computationally intensive, especially for large datasets.
- It may not be suitable when the error variance is correlated with the independent variables.
Final Words: LWLS is a valuable statistical technique for handling heteroskedasticity in linear regression models. By assigning appropriate weights to data points based on their estimated variance, LWLS provides more robust and efficient parameter estimates, ensuring more reliable conclusions from statistical analyses.
LWLS also stands for: |
|
All stands for LWLS |