What does PRPN mean in UNCLASSIFIED
PRPN (Parsing Reading Predict Networks) is a neural network architecture designed for reading comprehension tasks. It aims to improve the understanding of text by leveraging parsing and prediction techniques.
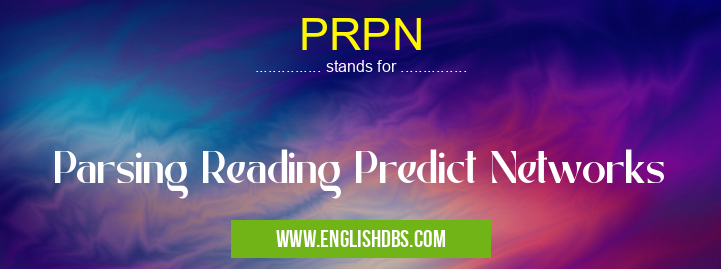
PRPN meaning in Unclassified in Miscellaneous
PRPN mostly used in an acronym Unclassified in Category Miscellaneous that means Parsing Reading Predict Networks
Shorthand: PRPN,
Full Form: Parsing Reading Predict Networks
For more information of "Parsing Reading Predict Networks", see the section below.
Key Features
- Parsing: PRPN incorporates a syntactic parser to understand the sentence structure and extract essential relationships between words.
- Prediction: It utilizes a prediction network to anticipate the next word or phrase in a sentence, based on the previous context.
- Integration: The parsing and prediction components collaborate to provide a comprehensive understanding of the text.
Advantages
- Improved Accuracy: PRPN's combination of parsing and prediction results in higher accuracy in reading comprehension tasks.
- Contextual Understanding: The parsing step helps the model understand the relationships within sentences, leading to a deeper contextual understanding.
- Word Prediction: The prediction network enables the model to anticipate upcoming words, facilitating a smoother reading process.
Applications
PRPN is primarily used in natural language processing tasks, particularly in:
- Machine Reading Comprehension: Answering questions based on provided text.
- Text Summarization: Condensing long text into a concise summary.
- Question Answering Systems: Developing conversational AI that can answer user queries.
Essential Questions and Answers on Parsing Reading Predict Networks in "MISCELLANEOUS»UNFILED"
What is PRPN (Parsing Reading Predict Networks)?
PRPN is an artificial intelligence (AI) model developed by Google AI that focuses on improving the ability of machines to read and understand text in a more comprehensive and context-aware manner. It combines techniques from natural language processing (NLP), machine learning, and deep learning to achieve this goal.
What are the key objectives of PRPN?
The primary goal of PRPN is to create a model that can parse, read, and predict the meaning of text with high accuracy. This involves accurately understanding the relationships between words, phrases, and sentences within a text, as well as predicting the semantic meaning and potential next words in a sequence.
How does PRPN work?
PRPN operates by utilizing a transformer-based encoder-decoder architecture. The encoder component of the model parses and encodes the input text into a compressed representation, capturing its syntactic and semantic information. This encoded representation is then passed to the decoder component, which generates a probability distribution over possible next words or sequences of words, predicting the continuation or completion of the text.
What are the potential applications of PRPN?
PRPN has a wide range of potential applications in various domains that rely on text understanding, such as:
- Machine translation: Enhancing the accuracy and fluency of translations between different languages.
- Summarization: Automatically generating concise and informative summaries of text documents.
- Question answering: Providing comprehensive and relevant answers to questions posed in natural language.
- Text classification: Accurately categorizing text documents into predefined classes or topics.
What are the advantages of using PRPN?
PRPN offers several advantages compared to traditional text processing methods:
- Contextual understanding: PRPN can capture the context and relationships within a text, enabling a more nuanced and comprehensive understanding.
- Predictive capabilities: It can predict the next words or sequences of words, facilitating tasks like language generation and completion.
- Scalability: PRPN can be trained on large datasets, leading to improved accuracy and robustness in handling diverse text inputs.
Final Words: PRPN is a powerful neural network architecture that enhances reading comprehension by integrating parsing and prediction techniques. Its key features, including syntactic parsing and word prediction, contribute to improved accuracy and contextual understanding in natural language processing tasks.