What does RFFR mean in FORESTRY
RFFR stands for Random Forest and Frequency Ratio. It is a machine learning algorithm used in feature selection and classification tasks.
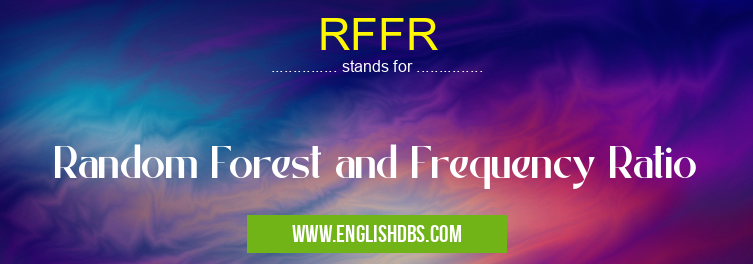
RFFR meaning in Forestry in Community
RFFR mostly used in an acronym Forestry in Category Community that means Random Forest and Frequency Ratio
Shorthand: RFFR,
Full Form: Random Forest and Frequency Ratio
For more information of "Random Forest and Frequency Ratio", see the section below.
Meaning in COMMUNITY
In the context of community detection, RFFR is a method for identifying community structures in complex networks. It combines the predictive power of Random Forest with the interpretability of Frequency Ratio.
Full Form
RFFR = Random Forest and Frequency Ratio
What does RFFR Stand for?
RFFR represents a combination of two techniques:
- Random Forest: A powerful ensemble learning algorithm for classification and regression.
- Frequency Ratio: A measure of association between a feature and a class label.
Working Principle
RFFR works by:
- Constructing a Random Forest classifier with n trees.
- Calculating the Frequency Ratio for each feature across the n trees.
- Selecting the top-ranked features based on their Frequency Ratio.
- Using these selected features for classification or predicting community membership.
Advantages
- Accuracy: Random Forest provides high predictive accuracy.
- Interpretability: Frequency Ratio allows for the identification of important features in community detection.
- Robustness: RFFR is less sensitive to noise and outliers compared to other feature selection methods.
Applications
RFFR is used in various applications, including:
- Community detection in social networks
- Gene expression analysis in bioinformatics
- Anomaly detection in cybersecurity
Essential Questions and Answers on Random Forest and Frequency Ratio in "COMMUNITY»FORESTRY"
What is Random Forest and Frequency Ratio (RFFR)?
RFFR is a machine learning technique that combines the power of Random Forest (RF) and Frequency Ratio (FR) to enhance feature selection and classification accuracy. RF is an ensemble learning method that builds multiple decision trees, while FR is a statistical measure that assesses the association between features and the target variable.
How does RFFR work?
RFFR follows a two-step process:
- Feature Selection: It uses FR to identify features that have a high correlation with the target variable.
- Classification: It trains an RF model using the selected features to predict the target variable.
What are the benefits of using RFFR?
RFFR offers several benefits:
- Improved Feature Selection: FR helps select the most relevant features, reducing overfitting and improving model performance.
- Enhanced Classification Accuracy: RF's ensemble approach enhances generalization ability and reduces prediction errors.
- Robustness: RF's bagging and randomization techniques provide robustness against overfitting and noise.
Where can RFFR be applied?
RFFR has applications in various domains, including:
- Predictive Analytics: Predicting outcomes such as customer churn, disease risk, or financial performance.
- Natural Language Processing (NLP): Text classification, sentiment analysis, and spam detection.
- Image Recognition: Object detection, image segmentation, and facial recognition.
Final Words: RFFR is an effective and efficient feature selection and classification algorithm for community detection in complex networks. It combines the benefits of Random Forest and Frequency Ratio to achieve high accuracy and interpretability.
RFFR also stands for: |
|
All stands for RFFR |