What does WDTW mean in UNCLASSIFIED
Windowed Dynamic Time Warping (WDTW) is an algorithm used to compare time series data and measure the similarity between them. It is a type of sequence comparison method that allows for the comparison of signals with different lengths or rates. WDTW was introduced by Stanfill and Waltz in 1986 and has been used ever since for various applications such as speech recognition, query by humming, bioinformatics, robotics, and many more.
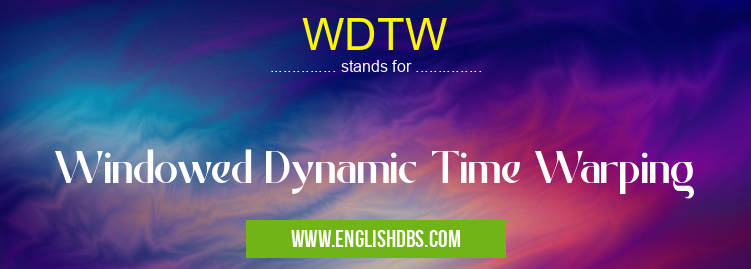
WDTW meaning in Unclassified in Miscellaneous
WDTW mostly used in an acronym Unclassified in Category Miscellaneous that means Windowed Dynamic Time Warping
Shorthand: WDTW,
Full Form: Windowed Dynamic Time Warping
For more information of "Windowed Dynamic Time Warping", see the section below.
Essential Questions and Answers on Windowed Dynamic Time Warping in "MISCELLANEOUS»UNFILED"
What is Windowed Dynamic Time Warping?
Windowed Dynamic Time Warping (WDTW) is an algorithm used to compare time series data and measure the similarity between them. It is a type of sequence comparison method that allows for the comparison of signals with different lengths or rates.
When was Windowed Dynamic Time Warping first introduced?
Windowed Dynamic Time Warping was first introduced by Stanfill and Waltz in 1986.
How is Windowed Dynamic Time Warping used?
Windowed Dynamic Time Warping can be used for various applications such as speech recognition, query by humming, bioinformatics, robotics, and many more.
Does WDTW provide a way to accurately measure similarity between time series data?
Yes, WDTW provides a way to accurately measure the similarity between two time series data points or signals of varying lengths or rates.
Is Windowed Dynamic Time Warping still in use today?
Yes, WDTW is still widely used today in various applications from speech recognition to robotics.
Final Words:
In conclusion, Windowed Dynamic Time Warping (WDTW) provides an efficient way to accurately measure the similarity between two time series data points or signals of varying lengths or rates. It was first introduced by Stanfill and Waltz in 1986 and has since been widely adopted for many applications like speech recognition, query by humming, bioinformatics, robotics and more.